Yes, customer churn rate prediction is possible. Here’s an evidence-based model for how to do it

We’re sometimes so preoccupied with acquiring new customers that we don’t invest enough in retaining the ones we have.
And in this economy, that often means we’re spending too much on efforts that don’t produce a high enough return.
When your customer acquisition costs are high, one of the smartest pivots you can make is to decrease your churn rate.
Churn rate is the percentage of your existing customers who don’t buy again within a given period—and it can reveal a lot of weaknesses about your business that need attention.
Churn is such an important business metric that researchers and academics have invested in creating formulas to predict it. We now have several models to work with, and some are better than others.
“In addition to standard segmentation capabilities, Klaviyo also offers advanced segmentation features such as predictive analytics, which can help ecommerce brands identify customers who are at risk of churning or are likely to make a future purchase,” says Lindsey Arellano, director of email and SMS at CURIO.
“This can help ecommerce brands target their email campaigns more effectively and increase customer lifetime value.”
Klaviyo also offers advanced segmentation features such as predictive analytics, which can help ecommerce brands identify customers who are at risk of churning or are likely to make a future purchase.
You’ll walk away from this article with a solid understanding of how to predict churn for your own business—and how you can use churn prediction to increase customer retention.
What is churn prediction?
Churn prediction is using data analysis, machine learning, and predictive modelling to forecast which customers are likely to stop buying—or simply never buy again. Churn prediction models help businesses proactively identify and retain customers who are most likely not to come back given time lapses that succeed the average next time to buy.
According to Klaviyo’s data science team, churn rates for average customers can be as high as 70%.
Low churn rates are better, so if you’re seeing churn prediction values of 50%, you’re in excellent shape with your customer base. But if you’re seeing churn prediction values above 75%, you have some decisions to make about retention strategies and prioritising the customers who are more likely to come back.
10 reasons customers churn: one-time payment models vs. subscription models
Before you can predict customer churn, you need to understand why customers churn in the first place. If you’re noticing a high churn rate, you’ll want to plug some holes in your business before fussing with churn prediction models.
Although there’s some overlap, one-time payment models and subscription models see different reasons for high churn rate. Here’s a list of common and not-so-common reasons for both business models:
One-time payment model churn reasons
1. Product dissatisfaction: It’s basic, it’s common, and it can be hard to fix—if your products don’t satisfy customers, your customers won’t buy again. If you’re seeing a high number of bad reviews, you may need to look into what aspects of your product design are deterring customers.
2. Shipping and fulfilment issues: Delays, damaged goods, or poor packaging can all lead to customer frustration and churn. Shipping and fulfilment issues are either a problem of communication—the customer’s expectations were set one way, but something else happened—or your business actually needs a shipping logistics review.
3. High prices: If one of your competitors starts offering a similar product at a lower price, customers may churn if they’re not loyal to your brand. Take care of customers by offering competitive pricing, the occasional discount, and customer appreciation promotions that make people feel special. And always communicate the unique value of your product—specifically for higher-ticket items.
4. Inaccurate product descriptions: If customers buy one thing and receive a product that doesn’t quite match its description, they may feel misled and opt not to buy again. Review your product description pages for accuracy and resist the urge to embellish outside the realities of what your product can do.
5. Lack of post-purchase support: Post-purchase support is especially important for products that require ongoing maintenance or education on optimal use. If customers buy a product like this and don’t receive proper guidance, they might not buy again.
6. Complex return/exchange policies: A restrictive or complicated return or exchange policy can discourage customers from making future purchases. It may sound counterintuitive at first, but an intuitive, convenient return or exchange process promotes retention in the long run by making sure customers get the products they actually want.
Subscription model churn reasons
1. Poor customer experience: Subscription models require ongoing customer support as a long-term relationship touchpoint. If your customer service doesn’t meet expectations, customers are more likely to cancel so they can feel cared for somewhere else.
2. Price increases: If you raise prices without proper and extensive communication about added value, your churn rate increase is likely to hit hard and fast. You may have solid economic reasons for raising prices, but you won’t want to do it without a long lead time, a sound communication plan, and strategies to cushion the blow when churn rates go up.
3. Lack of product customisation options: If customers feel like their subscription isn’t tailored to their preferences, they might churn. This depends on the product—razors, for example, may not require a lot of customisation, but a meal kit service might. If there’s room for customisation in your subscription plan, it’s worth offering more options to decrease churn.
4. Inflexible subscription plans: If customers feel bound by rigid subscription plans that don’t respect changes in lifestyle or circumstances, they may opt to leave if they can’t make the subscription work for themselves. Make sure to offer payment tiers, pause options, and easy renewal so you’re not losing customers permanently.
Why predict customer churn rate? Because you can prevent it.
It’s important to predict customer churn rate because you can do something about it. And depending on the nature of your business, it may be easier and cheaper to decrease your churn rate than acquire new customers.
Prediction leads to prevention. When you predict customer churn consistently and with a reasonable rate of accuracy, you can:
- Run targeted retention strategies: Send email and SMS offers to customers you identify as high risk.
- Save money on customer acquisition: When retention rates go up, you may be able to decrease spend on paid ads, for example.
- Increase customer satisfaction: Send preemptive customer support messages or educational resources to customers you identify as high risk.
- Proactively improve products: Start the long process of redesigning products before your churn rate becomes a mission-critical issue.
- Gain a competitive advantage: Predict churn when it’s unlikely your competitors are doing so, and address issues they may be missing.
Predicting churn rate is easier said than done
The thing is, predicting churn without the right models is as difficult as it is valuable.
And just because you have access to the customer data you need doesn’t mean it’s easy to extract in a way that’s useful.
For example, you may have a list of customer purchase events in your database. You can see that a certain number of purchases are associated with certain customers, and whether or not those customers viewed a product or opened an email after they made those purchases.
But it’s not easy to see the components of predictive data points in aggregate. When predicting churn, the sequence of purchase events and the time in between them are just as relevant as customer lifetime value (CLV) and the number of purchases themselves.
On top of this, accurate predictive analytics must be customised to how your business is actually operating. It’s one thing to learn churn formulas in business school as a general rule, but it’s another to predict actual outcomes using algorithms that are based on the realities of how your business operates.
Comparing individual customer actions to the behaviour of your entire customer population is crucial for accurate churn prediction you can act on.
How marketing automation platforms help predict churn
Marketing platforms use a variety of metrics and machine learning models to predict customer churn, but the key metric they lean on is CLV. CLV is calculated by considering the customer’s average purchase value, average purchase frequency, and average lifespan with your business.
To forecast CLV to churn risk, you need access to a lot of ecommerce data points. This access allows you to compare individual CLV to a variety of metrics within your entire population of customers.
This is where many models, including highly cited academic models, for predicting churn fall short—researchers at academic institutions only have access to publicly available data, whereas Klaviyo uses a ton of real-time data to refine its churn prediction models over time.
Here, we describe how Klaviyo predicts churn, then compare it to models in academic literature in more detail.
Klaviyo’s churn risk model in action
Klaviyo’s churn risk model begins with some rules. These criteria are in place to make sure the churn prediction is as accurate as possible:
- The business has at least 500 customers who have placed an order. This is the sample size required to compare individual behaviour to a large enough segment of your overall customer behaviour.
- The business has at least 180 days of order history and has orders within the last 30 days.
- The business has at least some customers who have placed 3 or more orders.
If your business meets the data requirements, you’ll be able to see a visualisation that looks like this:
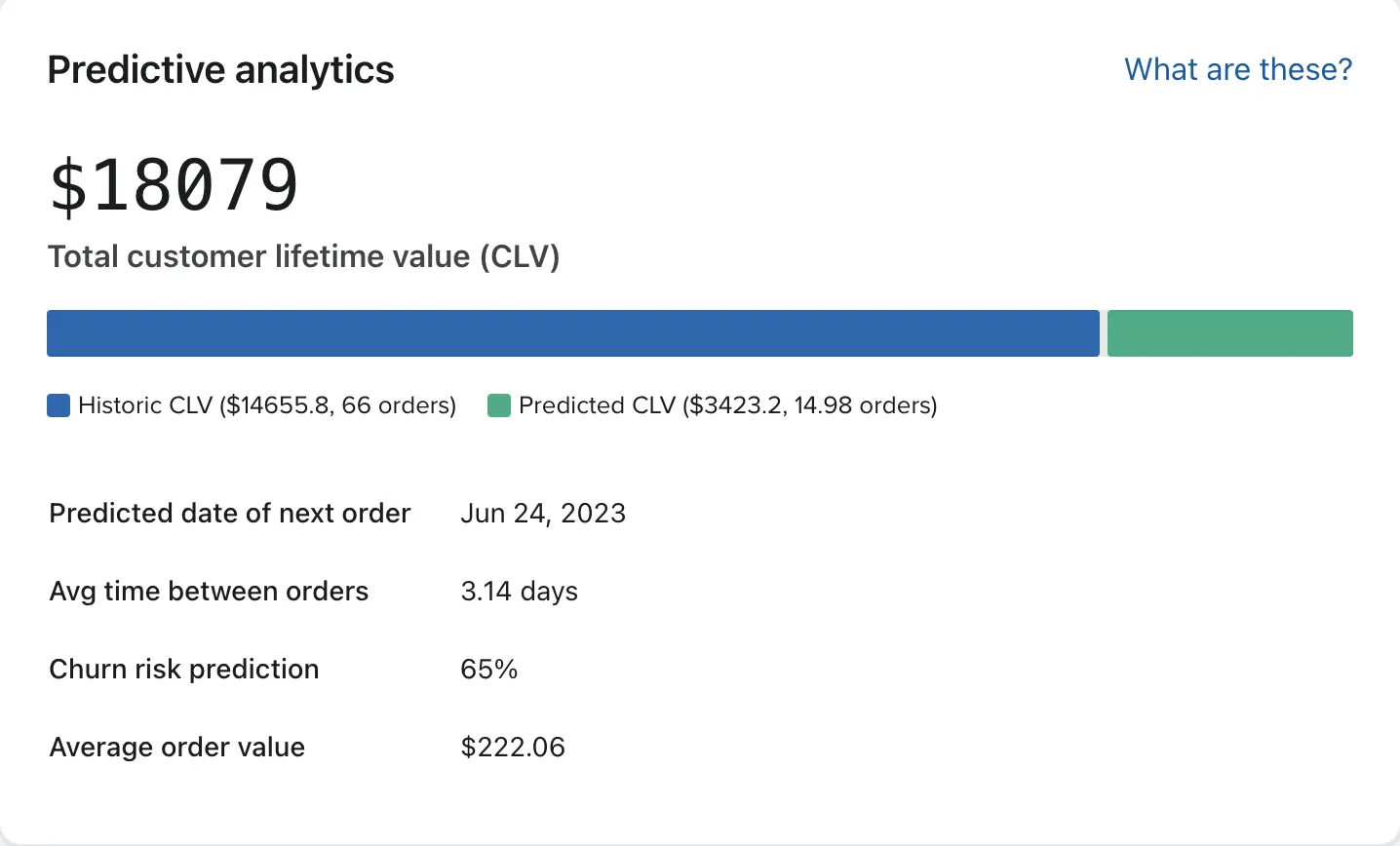
The coloured bar represents a customer’s total CLV. The blue section represents Historic CLV—what the user has spent on your offerings so far. The green section represents Predicted CLV—what we expect them to spend with your brand in future. Below this, you can find the expected date of next order, the average time between orders, average order value, and the churn risk prediction at the moment you’re looking at the graph.
Klaviyo’s model is trained on the history of your customers. To score a customer’s individual churn risk, the model incorporates the following in addition to CLV:
- Number of orders
- Time between orders
- The most recent order
Generally, when a customer purchases, they are at a low risk of churn. Then, as time passes, their churn risk gradually increases as the time since last purchase lengthens.
But the model isn’t that simple—it also learns over time, based on how frequently a customer actually purchases. For example, a customer might wait 100 days between orders. Over time, their risk of churning might remain lower for longer than for a customer who typically waits, say, 50 days between orders, according to the business’s unique customer churn prediction model.
Klaviyo’s model is further refined by comparing individual purchase behaviour to similar actions across your business’ customer population, especially when it comes to the behaviours that lead to repeat purchases.
As a result, every company has a unique churn model. Klaviyo doesn’t use customer population data from other companies to inform churn risk because it wouldn’t necessarily apply to that business.
How do we know the new Klaviyo model performs better than the academic models?
Generally, the larger the data set, the more accurate the results.
Klaviyo’s model is trained on 700 data sets, whereas the academic models we researched were trained on 3.
And without a minimum data requirement, the academic model uses sample sizes that are too small to reflect reality. Publicly available data on customer churn rates is limited and may not apply to the vast majority of businesses.
Then, there’s the problem of…Poisson.
No, we’re not talking about fish. In data analysis, a Poisson distribution represents the probability of an event happening a certain number of times within a given interval of time. When Klaviyo’s data analysis team first began to examine the academic model of churn prediction, they noticed a massive error: the model used a Poisson distribution, but the data feeding into it was not shaped like Poisson data.
As a result, customers were assigned a low predicted churn rate—because some of them were predicted to make a purchase within the next 50K years. The academic model was mixing up the time recurrence prediction and the churn prediction because the real data did not match the Poisson distribution that was required.
The academic model is overly optimistic, assigning a medium 40-70% probability of churn to a large number of customers. But when we compare these predictions to real customers in Klaviyo, we see that 88-97% of these medium-risk customers churn—showing the academic model can’t differentiate between medium-risk customers and high-risk customers.
What next? 6 ways to lower customer churn rate
Churn analysis isn’t useful unless you take action. If you’re seeing a high number of customers with a churn prediction value of 75% or higher, that’s your cue to plug some holes in your customer experience. Here’s a few use cases you can start with:
1. Continuous product improvement
If you’re seeing high churn prediction values and an increasing number of bad reviews, it’s a sign that marketing alone won’t repair your churn problem.
Start collecting customer feedback through surveys, customer support conversations, and social media. Ask specific questions about where your products are coming up short, and be explicit about your desire to work on product optimisation. Once you collect enough qualitative data sources, start the hard work of changing your products to meet the needs of your customers.
2. Targeted win-back email marketing campaigns
A win-back email is great for customers whose churn prediction is creeping up but hasn’t yet reached critical levels (90%+). Win-back email campaigns are automated emails you can set to trigger when churn prediction reaches a certain point. That means you can write them once, review them on occasion, and let automation take care of the rest.
If you need some inspiration, check out this example from Girlfriend Collective. They ditch a lot of the flash in favour of a minimalist email that provides a simple, time-based incentive.
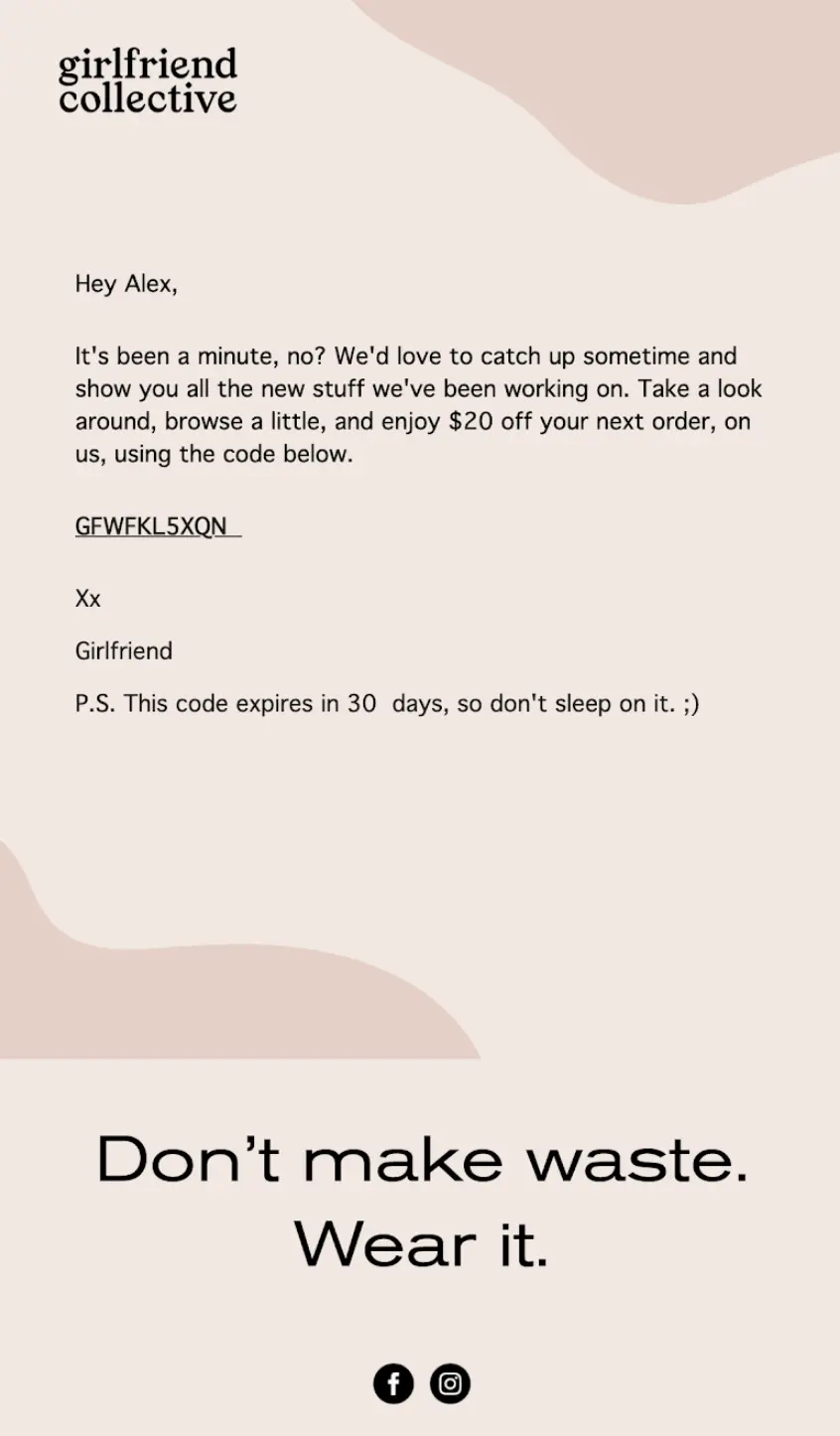
3. Loyalty programs with special offers
Depending on your category, a loyalty program may be the most lucrative low-hanging fruit you can rely on to decrease customer churn rate. Here are the top industries in which consumers actively participate in loyalty programs:
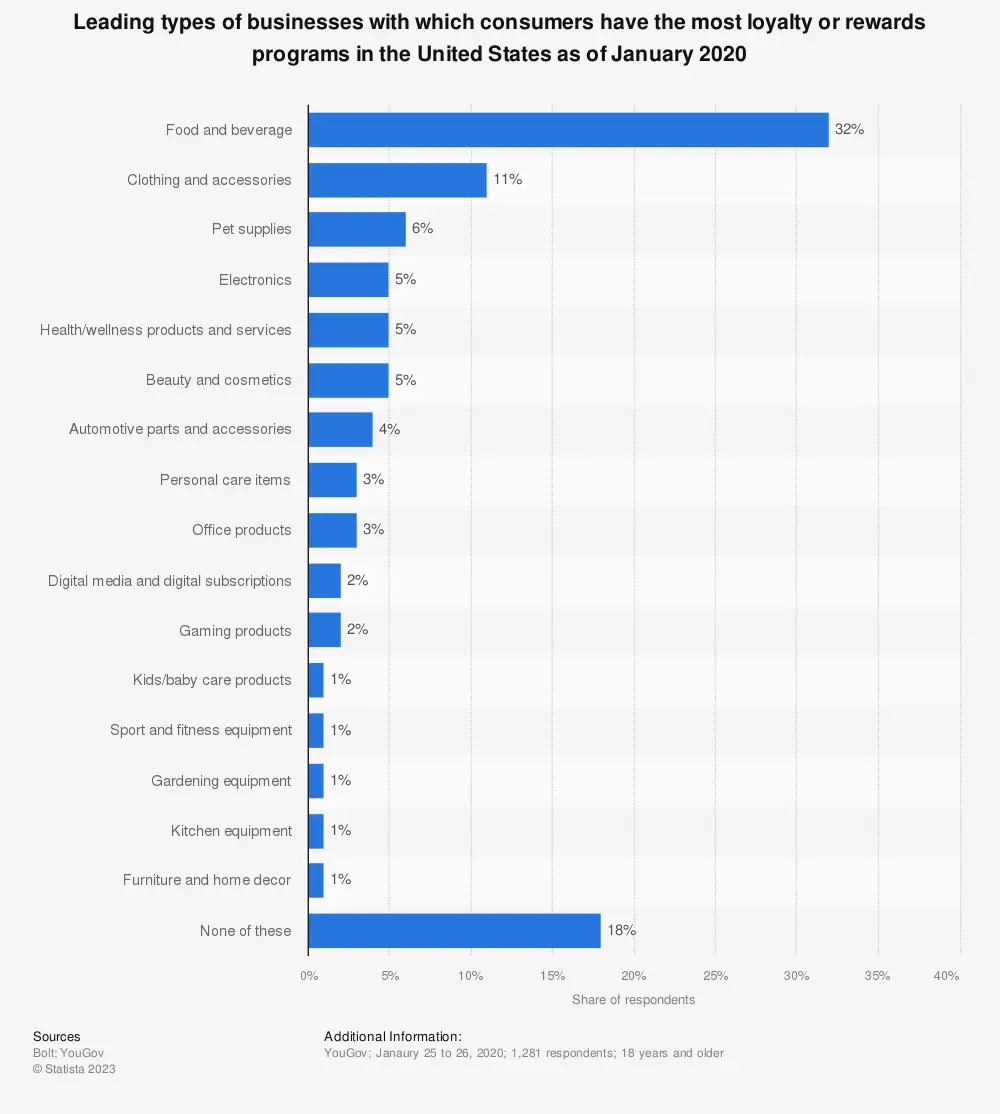
If you don’t have a loyalty program and want to test one, we recommend launching to customers at a higher risk of churning. If you see your overall churn rate decrease a few months post-launch, it could be your sign to launch the program more widely.
4. Proactive customer support
Chatbots, automated support systems, and proactive notifications are all tools that can automate proactive customer service. As conversational AI gets better, you may be able to invest in additional customer support without blowing your budget.
“To reduce churn, it’s important to provide exceptional customer service and promptly address any issues or concerns,” says Arellano. “Make it easy for customers to contact you and provide various channels for them to reach out, such as email, phone, or live chat.”
5. Educational content and resources
If your product requires assembly or ongoing maintenance, educational content and resources as post-purchase support are excellent ways to reduce churn. Even if your product doesn’t require assembly, consider sending instructional videos about how to get the most use out of the product.
For example, Competitive Cyclist sends every customer an assembly instructional video. Bike assembly can feel overwhelming even for the most seasoned cyclist, so this is just one way Competitive Cyclist uses educational content to take care of their customers—and make sure they’re top of mind when someone needs tools, accessories, or additional parts.
6. Social proof reminders
Sometimes customers who are about to churn just need a reminder that other people love your products. Returning to our win-back campaign suggestion, an option for those emails is to include a positive quote from a loyal customer. This example from Printfresh shows what this can look like at the end of an email.
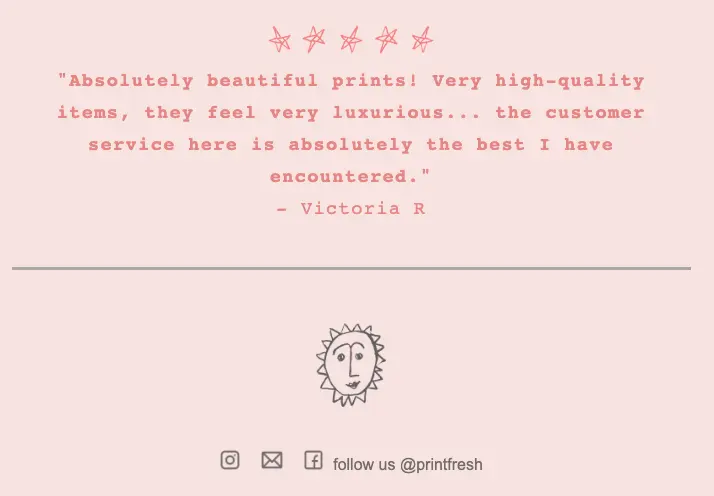
Keep more customers coming back with churn prediction analytics
When you can predict churn, you can prevent churn.
And with an accurate and reliable predictive analytics model, you can be sure that you’re messaging the right customers, at the right time, with the most relevant messaging possible.
Make sure your at-risk customers don’t pass up your brand without giving it a second chance—and reduce customer attrition for your brand.
FAQs about churn rate
How to start using churn prediction?
Predictive analytics for ecommerce businesses are improving at breakneck speed. Use Klaviyo’s built-in AI technology to help you scale and improve your marketing strategy.
How can brands calculate churn rate?
To calculate churn rate, divide the number of customers who have churned during a period of time by the total number of customers at the beginning of that period, then multiply by 100 to get a percentage.
What are the main reasons for customer churn?
The main reasons for customer churn include:
- Product dissatisfaction
- Shipping and fulfilment issues
- High prices
- Inaccurate product descriptions
- Lack of post-purchase support
- Complex return/exchange policies
- Poor customer experience
- Price increases
- Lack of product customisation options
- Inflexible subscription plans
How does churn rate impact revenue?
Churn rate represents lost revenue because it’s the rate at which customers are leaving the business. Higher churn rates mean a large number of customers aren’t coming back, but it also means they’re not spreading positive word of mouth about your business.
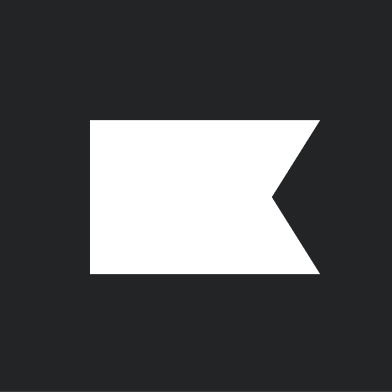